51 | Add to Reading ListSource URL: www.aaai.orgLanguage: English - Date: 2006-01-11 06:46:07
|
---|
52![572 IEEE TRANS-WTIONS ON AUTOMATIC CONTBOL, AUGUST[removed]can be controlled by judicious choice of k. By choosing k = 1, Aro = 0 so that the transient term is monot,onically decreasing from 572 IEEE TRANS-WTIONS ON AUTOMATIC CONTBOL, AUGUST[removed]can be controlled by judicious choice of k. By choosing k = 1, Aro = 0 so that the transient term is monot,onically decreasing from](https://www.pdfsearch.io/img/17ea672a56f791c6415acf76d0b54239.jpg) | Add to Reading ListSource URL: eprint.iitd.ac.inLanguage: English - Date: 2006-04-04 16:30:26
|
---|
53![572 IEEE TRANS-WTIONS ON AUTOMATIC CONTBOL, AUGUST[removed]can be controlled by judicious choice of k. By choosing k = 1, Aro = 0 so that the transient term is monot,onically decreasing from 572 IEEE TRANS-WTIONS ON AUTOMATIC CONTBOL, AUGUST[removed]can be controlled by judicious choice of k. By choosing k = 1, Aro = 0 so that the transient term is monot,onically decreasing from](https://www.pdfsearch.io/img/54f80f1c7c16f55280866a3ef50fa32f.jpg) | Add to Reading ListSource URL: eprint.iitd.ac.inLanguage: English - Date: 2006-04-04 16:30:26
|
---|
54 | Add to Reading ListSource URL: fab.cba.mit.eduLanguage: English - Date: 2014-04-29 10:51:08
|
---|
55 | Add to Reading ListSource URL: www.stat.berkeley.eduLanguage: English - Date: 2010-05-04 01:48:29
|
---|
56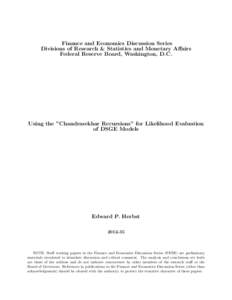 | Add to Reading ListSource URL: www.federalreserve.govLanguage: English - Date: 2012-06-29 13:10:58
|
---|
57 | Add to Reading ListSource URL: trec.nist.govLanguage: English - Date: 2002-02-12 09:50:34
|
---|
58 | Add to Reading ListSource URL: msa.medicine.iu.eduLanguage: English |
---|
59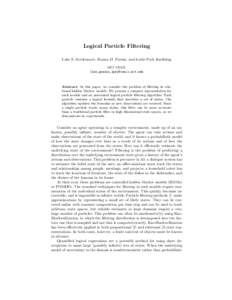 | Add to Reading ListSource URL: people.csail.mit.eduLanguage: English - Date: 2007-07-22 10:19:15
|
---|
60 | Add to Reading ListSource URL: www.stat.columbia.eduLanguage: English - Date: 2013-09-18 03:45:49
|
---|